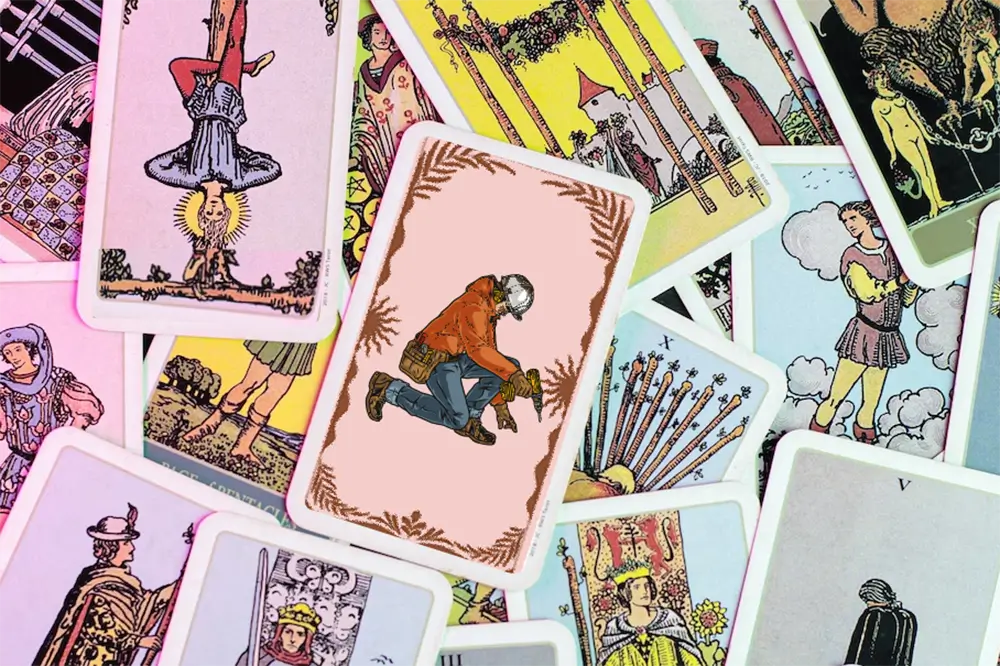
Lösungsansätze für vorausschauende Wartung von Maschinen
Produzierende Unternehmen in Deutschland investieren laut statistischem Bundesamt über 50 Milliarden Euro pro Jahr in Maschinen und Anlagen. Entsprechend hoch sind die Bemühungen, Ausfälle zu verhindern. Predictive Maintenance avanciert deshalb zum zentralen Bestandteil der Industrie 4.0.
Produktionsketten werden immer komplexer. Damit sind sie deutlich häufiger und stärker von Mängeln betroffen. Eine veraltete Instandhaltungsstrategie kann die Produktionskapazität eines Standorts um bis zu 20 Prozent senken. Immer mehr Unternehmen setzen deshalb auf den Einsatz von Predictive Maintenance. Bei der “vorausschauenden Wartung” wird, im Gegensatz zur vorbeugenden Wartung, auf die Vorhersage von Wartungsbedarf bei Maschinen und Anlagen vertraut, um Ausfallzeiten zu minimieren und Wartungsaufwand zu verringern. Hierfür kommen Sensordaten zum Einsatz, mit denen viele Maschinen bereits von Werk aus gerüstet sind.
Vorbeugende Wartung bedeutet, Instandhaltungsmaßnahmen nach vordefinierten Kriterien durchzuführen, zum Beispiel alle 7.000 Betriebsstunden ein Leuchtmittel zu wechseln. Vorausschauende Wartung hingegen bedeutet, dass anhand von Kennzahlen und den Beziehungen zwischen diesen Kennzahlen der Wartungsaufwand automatisch bestimmt und für geringe Ausfallzeiten und hohe Maschineneffektivität optimiert wird. Predictive Maintenance kann im Vergleich zu vorbeugender Wartung die Verfügbarkeit von Produktionsanlagen um 10 bis 20 Prozent steigern, während die Instandhaltungskosten um 5 bis 10 Prozent sinken.
Wie funktioniert Predictive Maintenance?
Kurz gesagt bedeutet Predictive Maintenance, oder vorausschauende Wartung in der Industrie 4.0, dass der Wartungsbedarf von Anlagen und Maschinen automatisch und individuell bestimmt wird. Dabei nähert sich ein Algorithmus immer weiter dem optimalen Zeitpunkt für eine Wartung an, um Ausfallzeiten zu minimieren und Kosten zu sparen. Der optimale Abstand zwischen zwei Wartungen kann sich dabei zwischen Maschinen des gleichen Modells stark unterscheiden, da jede Maschine einzeln analysiert wird.
Für vorausschauende Wartung lernt ein Algorithmus aus Daten bestimmte Muster, die auf einen Wartungsbedarf hindeuten. Sensordaten kommen bei nahezu jeder Lösung zum Einsatz, aber immer mehr Systeme verwenden zusätzliche Bilderkennung oder externe Informationen wie die Art des verwendeten Materials. Beispielsweise können Ermüdungen im Material durch eine Veränderung des Klangs festgestellt werden. Sensoren erfassen dann Tonfrequenzen, die für den Menschen nicht hörbar sind. Dadurch lassen sich Anzeichen für einen bevorstehenden Defekt bis zu zwei Wochen früher erkennen.
Erhebung von Sensordaten
Die <a href="https://taod.de/services/bi-und-data-analytics-consulting“ data-webtrackingID="blog_content_link" > Datenanalyse </a> erfolgt über Sensordaten. Bereits ein Drittel aller genutzten Maschinen in Deutschland sind mit Sensoren ausgestattet. Gerade neue Modelle erhalten immer häufiger integrierte Sensoren. Daten wie Temperatur, Betriebsdauer, Drehzahlen, Druck und Vibration werden für die vorausschauende Wartung besonders häufig benutzt.
Grundsätzlich gilt: Mit großen Datenmengen wird die Bestimmung von Wartungsaufwänden deutlich zuverlässiger. Viele mittelständische Unternehmen, die bisher nur wenige Berührungspunkte zu Big Data und künstlicher Intelligenz hatten, klagen über Hürden in der Implementierung, verfügen nicht über die nötigen Kompetenzen im Unternehmen und sehen hohe Kosten auf sich zukommen. Doch diesen Herausforderungen der Industrie 4.0 lässt es sich mit der richtigen Langzeitstrategie kompetent begegnen.
Herausforderungen und Lösungen für erste Projekte mit Predictive Maintenance
Jeder Betrieb agiert unter individuellen Umständen, Rahmenbedingungen, Anforderungen und Zielsetzungen. Es sind aber auch einige Herausforderungen zu beobachten, denen regelmäßig in Predictive-Maintenance-Projekten beizukommen ist. Diese Herausforderungen können kategorisiert und dann systematisch gelöst werden.
Datenerfassung
Daten bilden die Grundlage für jeden lernenden Algorithmus. Doch zunächst einmal müssen diese erfasst werden. Datenerfassung und Data Management funktionieren, wenn Daten gesammelt, übertragen und gespeichert werden. Immer mehr Anlagen verfügen hierfür über integrierte Sensoren, die Daten automatisch erfassen.
Lösung für Datenerfassung
Die Datenerfassung ist eine Zusammensetzung aus mehreren Teilprozessen. Jeder dieser Teilprozesse sollte den Best-Practice-Standards unterliegen. Diese Standards bieten zwei Vorteile: Wiederverwendbarkeit und Robustheit. Funktionierende Teilschritte können mit leichten Anpassungen für andere Fälle verwendet werden. Dadurch sinkt der Aufwand für den Aufbau und die Weiterentwicklung von Systemen zur Datenerfassung und diese bleiben trotzdem flexibel. Gleichzeitig wird ein hoher Qualitätsstandard sichergestellt. Die einzelnen Komponenten sind vielfach praxiserprobt und so optimiert, dass sich der schnelle Aufbau des Systems mit hoher Leistungsfähigkeit vereinen lässt. Die Auswahl der konkreten Werkzeuge ist dabei von größter Relevanz. Erfahrung mit <a href="https://taod.de/tech“ data-webtrackingID="blog_content_link" > geeigneten Technologien </a> kann den Aufbau der Datenerfassung immens beschleunigen und langfristige Kosten auf ein Minimum reduzieren.
Datenaufbereitung
Die gesamte Datenerfassung und alle Teilschritte sind darauf optimiert, einen reibungslosen und schnellen Ablauf zu ermöglichen. Dafür werden Daten verpackt und komprimiert. Daten können dadurch sehr flexibel verwendet werden, bei gleichzeitig geringen Kosten für die Speicherung und den Transfer. Damit ein Algorithmus aus Daten lernt, müssen diese aber in ein anderes Format gebracht werden. Hinzu kommt, dass Daten immer fehlerhaft oder unvollständig sein können. Eine hohe Datenqualität ist die Voraussetzung für zuverlässige Künstliche Intelligenz. Die sogenannte “Rule of Ten” ist eine der wichtigsten Regeln in der Arbeit mit Daten. Sie besagt, dass Arbeitsschritte mit hochwertigen Daten um 90 % günstiger sind als mit fehlerhaften Daten, weil sie deutlich schneller erledigt werden und viel seltener Fehler produzieren.
Lösung für Datenaufbereitung
Auch für die Sicherstellung einer hohen Datenqualität ist die Wahl der richtigen Werkzeuge entscheidend. Dabei kommt es stark auf die Entwicklung effektiver Prozesse zur Kontrolle der Datenqualität an. Ein effektiver Kontrollprozess liefert Erkenntnisse darüber, welche Qualitätsmängel bestehen und wie diese behoben werden können.
Der Einsatz von <a href="https://www.taod.de/services/data-engineering-consulting“ data-webtrackingID="blog_content_link" > Engineering </a> Best Practices sorgt dafür, dass die Datenqualität auf einem hohen Level gehalten werden kann. Tools wie <a href="https://www.taod.de/tech-beratung/dbt-labs“ data-webtrackingID="blog_content_link" > dbt </a> machen die Umsetzung von Best Practices in der Datenaufbereitung auch für junge, kleine Data-Teams möglich. Richtig konfiguriert sorgt dbt dafür, dass Mängel erkannt werden, schon bevor sie tatsächlich auftreten.
Datenkombination
Künstliche Intelligenz basiert darauf, dass Regeln und Muster in Daten erkannt werden. Damit das passieren kann, müssen diese Muster in den Daten enthalten sein. Einer der wichtigsten Erfolgsfaktoren für Predictive-Maintenance-Projekte ist die Anreicherung von Daten. Gewisse Regelmäßigkeiten können erst durch die Ergänzung weiterer Informationen aufgedeckt werden.
Lösung für Datenkombination
Die Kombination von Daten aus verschiedenen Quellsystemen wirkt im ersten Moment wie ein zusätzlicher Aufwand. Tatsächlich ist es so, dass dieser Schritt die Kosten für Predictive Maintenance sogar senken kann und zusätzliche Flexibilität ermöglicht.
Um die sinnvollen Möglichkeiten zur Datenanreicherung zu identifizieren, ist die fachliche Erfahrung im Einsatz der Anlagen und Maschinen von höchster Wichtigkeit. Durch den Austausch von KI-Entwickelnden und Produktionsmitarbeitenden werden mögliche Zusammenhänge direkt ausgetauscht und können in kürzester Zeit auch in das Predictive-Maintenance-System eingebunden werden. Alle verwendeten Informationen werden nach ihrem Mehrwert beurteilt, indem die Verbesserungen der Performance und Aufwände gegenübergestellt werden. Diese Gegenüberstellung erfolgt automatisch und steht dauerhaft für weitere Optimierungen und für das Controlling zur Verfügung.
Erfolgsfaktoren für Projekte mit Predictive Maintenance
Der Erfolg von Projekten mit Predictive Maintenance ist durchaus vorbestimmbar, wenn wichtige Grundvoraussetzungen erfüllt sind. Im Bereich der <a href="https://taod.de/services/bi-und-data-analytics-consulting/“ data-webtrackingID="blog_content_link" > klassischen Datenanalyse </a> müssen Unternehmen deshalb dringend ihre Hausaufgaben machen. Denn eine Faustregel gilt es ganz dringend auch in der Industrie 4.0 zu beachten: Erst Data Analytics, dann Data Science.
Transparenz des Wartungsaufwands
Selbst wenn eine ausreichende Datenbasis vorhanden ist, bedeutet das nicht, dass diese auch gesehen werden. Damit die Daten nicht nur auf dem Server oder in der Cloud gesammelt, sondern auch sichtbar werden, müssen sie visualisiert und allen Verantwortlichen zugänglich gemacht werden. Die Umsetzung kann über Dashboards, automatisierte Mails sowie die Integration in bestehende Kommunikationswerkzeuge wie Slack oder Trello erfolgen. Die Arbeit eines Produktionsleitenden oder technischen Asset Managers wird mit diesen, oft in Echtzeit ausgelieferten Daten, zuverlässig ergänzt. <a href="https://taod.de/services/bi-und-data-analytics-consulting/“ data-webtrackingID="blog_content_link" > Professionelle Datenanalyse </a> über einen Modern Data Stack gilt deshalb als Erfolgsgarant für Predictive Maintenance.
Vorhersage des Wartungsaufwands
Wann und wie viel Aufwand für die Wartung von Maschinen entsteht, lässt sich mithilfe Künstlicher Intelligenz und unter Verwendung entsprechender Machine-Learning-Algorithmen vorhersagen. Durch Algorithmen werden Maschinen erst lernfähig. Wichtig ist, dass die gemessenen Daten reibungslos überwacht werden, um Systemdiagnosen zu erstellen, Abweichungen sowie mutmaßliche Probleme automatisch zu erkennen und eine Prognose über die nutzbare Restlebensdauer abzugeben. Welcher AI-Algorithmus zum Einsatz kommt, ist abhängig von der überwachten Maschine. Unterschieden wird zwischen Klassifizierung und Anomalie-Erkennung. Während die Klassifizierung eine sinnvolle Möglichkeit für die Überwachung von Maschinen mit hoher Ausfallquote ist, kommt die Anomalie-Erkennung bei Geräten zum Einsatz, die kaum fehleranfällig sind. Deren Überwachung ist um ein Vielfaches komplexer, da es hier selten Anzeichen für Ausfälle gibt.
Rohdaten aus Sensoren
Für Predictive Maintenance werden Sensoren genutzt, die bereits in der Anlage integriert sind oder mit geringem Aufwand angebracht werden können. Bei den zu erfassenden Daten wird unterschieden zwischen notwendigen und zusätzlichen Daten. Notwendige Daten sind Voraussetzung für Predictive Maintenance. Zusätzliche Daten verbessern die Qualität der Vorhersagen, sind aber kein Muss. Die wichtigsten zu erfassenden Daten sind Infos über Ausfälle in der Vergangenheit, Temperatur, Betriebsdauer, Drehzahlen, Druck, Vibration und indirekte Parameter wie zum Beispiel das verwendete Material oder Zulieferer. Welche davon notwendig sind, wird im Einzelfall entschieden.
Ablauf eines Projekts für Predictive Maintenance
Ein Predictive-Maintenance-Projekt benötigt sorgfältige Vorbereitung, kann dann aber schnell auf Spur gebracht werden. Im Grunde lässt es sich in die drei Phasen Konzept, MVP und Analyse aufteilen:
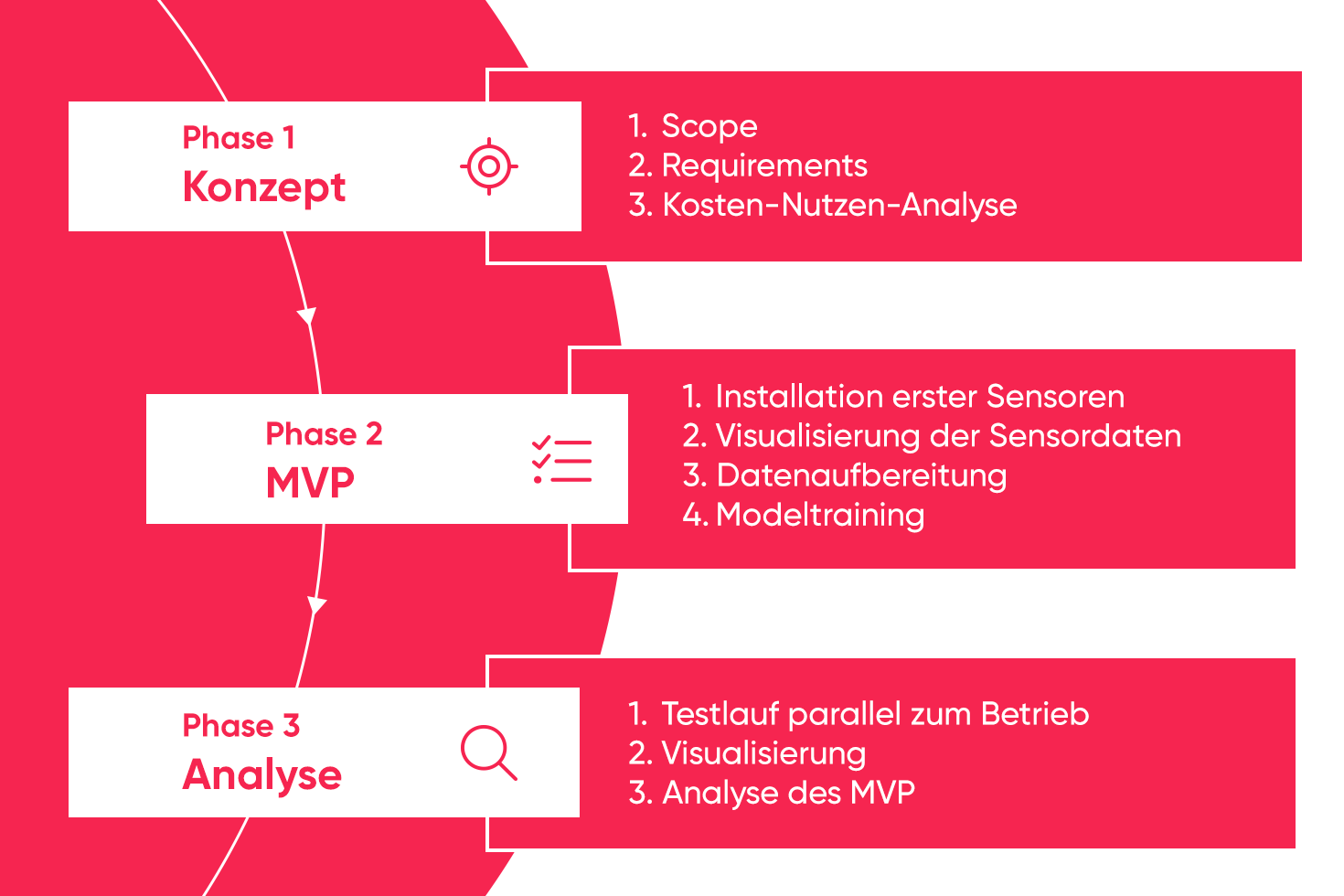