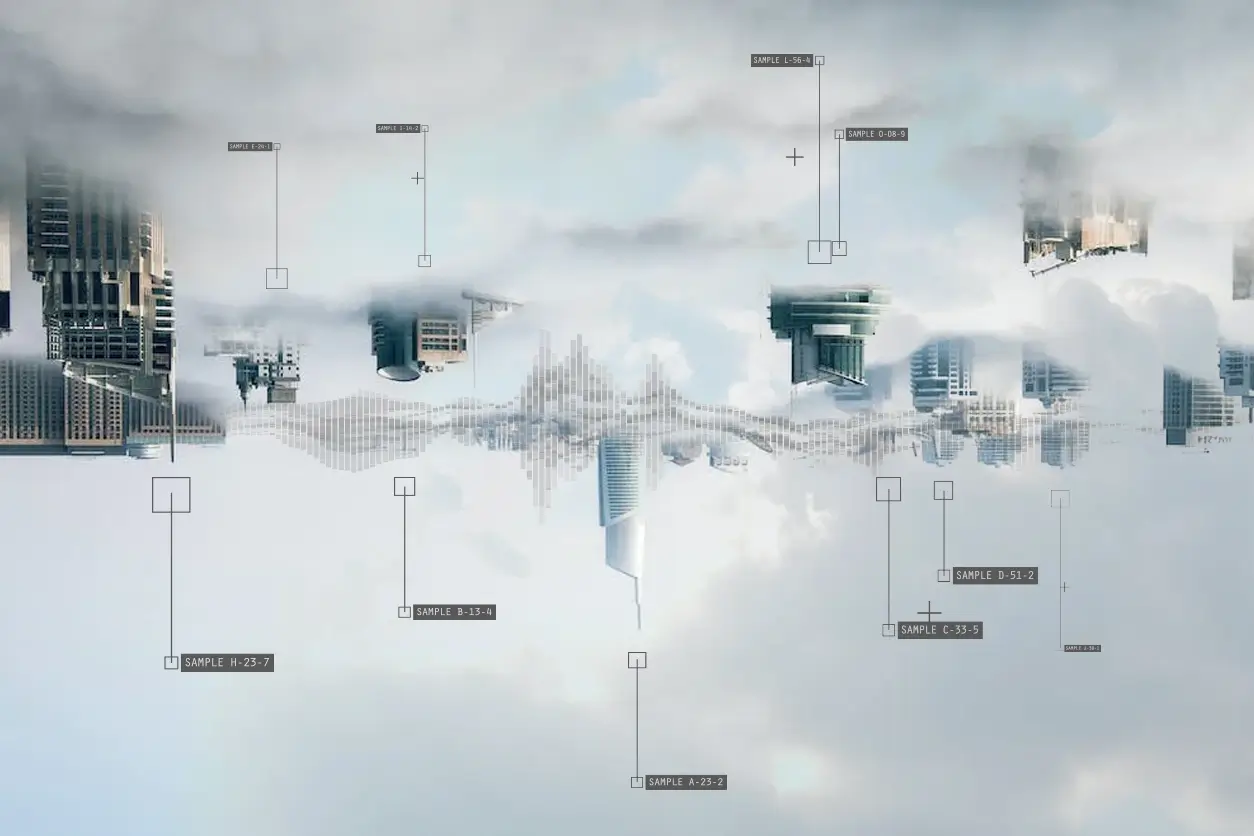
Vollintegrierte Lösungen für jedes Data-Science-Projekt
Manche Data-Science-Projekte schaffen es nie weiter als bis zur Prototyp-Phase. Und das, obwohl die Performance der entwickelten Modelle zufriedenstellend ist. Das liegt vor allem daran, dass Data Science als Entwicklung nicht oder kaum in das bestehende Datenökosystem und die Prozesse des Unternehmens integriert ist. Wird Machine Learning jedoch von Beginn an in der Cloud verortet, findet ein Projekt in Data Science zu nachhaltigem Erfolg.
Alles dreht sich um Daten und um Analysen
Ist die Rede von Machine Learning in der Cloud, werden in einem Atemzug eigentlich immer die drei Public-Cloud-Anbieter Google, AWS und <a href="https://www.taod.de/tech-beratung/microsoft-azure“ data-webtrackingID="blog_content_link" > Azure </a> genannt. Diese Dienste sind nicht nur etablierte Hyperscaler, sondern bieten vor allem einen schnellen und kostengünstigen Einstieg in die für datengetriebene Projekte benötigten Cloud-Technologien. Data Scientists nutzen ihre Machine-Learning-Verfahren für vielfältige Anwendungsszenarien direkt in der Cloud und führen diese dort aus. Der Einstieg in die Cloud mit einem Data-Science-Projekt gelingt also im Kleinen und kann nach Bedarf hochskaliert werden. Angebot, Kosten und Nutzerfreundlichkeit unterscheiden sich teilweise markant unter den Anbietern, so dass vor Auswahl einer geeigneten Plattform eine ausführliche Bewertung der einzelnen Features vorgenommen werden sollte.
Machine Learning für Data Science in der Cloud bietet einen einfachen Einstieg in die Materie und ermöglicht eine simple Übergabe an technisch nicht affine Menschen, denn für Drag & Drop sind keine Programmierkenntnisse nötig. Trotzdem bleibt die Umgebung flexibel, denn individuelle Speziallösungen können leicht durch Python Code integriert werden. Die Modellstruktur bleibt stets übersichtlich visualisiert.
Integration von der Datenbank bis zum Dashboard
Dass die Data-Science-Entwicklung direkt in der Cloud stattfindet, bedeutet von Anfang an eine unmittelbare Integration in den Data Workflow der Organisation. Das hat mehrere Vorteile: Einerseits knüpft das Modell direkt an die Datenbanken an und vermeidet somit Umwege durch die Zwischenspeicherung auf Endgeräten der Entwicklung. Das schlägt sich insbesondere bei großen Datenmengen in der Übertragungsgeschwindigkeit, aber auch in den Kosten nieder. Gleichzeitig ist eine direkte Übertragung, die gegebenenfalls sogar serverintern stattfinden kann, weniger anfällig für Angriffe durch Schadsoftware.
Ebenso wie der Daten-Input sind auch die Ergebnisse des Machine-Learning-Modells direkt anschlussfähig für die Verarbeitung in weiteren Schritten, wie beispielsweise der Visualisierung in <a href="https://www.taod.de/tech-beratung/power-bi“ data-webtrackingID="blog_content_link" > Power BI </a> oder <a href="https://www.taod.de/tech-beratung/tableau“ data-webtrackingID="blog_content_link" > Tableau </a> Dashboards. Damit ist eine direkte Integration der Data-Science-Maßnahmen in die Arbeitsabläufe im Unternehmen sichergestellt. Data-Science-Lösungen müssen stets von Beginn an im Gesamtkontext der Organisation gedacht und entwickelt werden.
Skalierbarkeit, Flexibilität, Verfügbarkeit
Die allgemeinen Vorteile der Cloud werden im Kontext von Data Science und vor allem Data-Science-Projekten zum zusätzlichen Game Changer. So steht für die bisweilen riesigen Datenmengen die volle Bandbreite des Cloud-Anbieters zur Verfügung, ganz unabhängig von der firmeneigenen Infrastruktur, die nur mit hohem Kostenaufwand gleiche Leistung erbringen kann. Und auch bei schnell wechselnden Anforderungen, wie zum Beispiel starkem Wachstum oder unsteten Belastungsspitzen, liefern <a href="https://www.taod.de/services/data-engineering-consulting“ data-webtrackingID="blog_content_link" > Cloud-Lösungen </a> für Data-Science-Projekte eine hohe Skalierbarkeit und Flexibilität. Diese abstrakten Vorteile sind im konkreten Anwendungsfall von unschätzbarem Wert.
Hierzu ein Beispiel: Eine Einzelhandelskette möchte mittels Machine-Learning-Algorithmen Vorhersagen über Lagerbestände und Produktnachfragen tätigen. Ziel ist es, die tägliche Lieferkette ideal auf die Nachfrage der Kunden und Kundinnen abzustimmen. Je nach Angebotsvielfalt können dabei verschiedenste Daten verarbeitet werden. Die Cloud-Architektur stellt dabei nicht nur die Verfügbarkeit der nötigen Bandbreite sicher, sondern auch, dass Ausfälle der so kritischen Datenverarbeitung im Notfall auf andere Server verteilt werden können. Das Risiko von Lagerengpässen und den damit verbundenen Umsatzeinbußen wird somit auf ein Minimum reduziert.
Datenschutz in der Cloud
Auf <a href="https://www.taod.de/tech-beratung/microsoft-azure“ data-webtrackingID="blog_content_link" > Azure basierende Cloud-Lösungen </a> sind DSGVO-konform. Mit der richtigen Konfiguration kann die Architektur so konstruiert werden, dass sensible Daten die EU nicht verlassen.
- Freie und große Auswahl des Serverstandorts ermöglicht DSGVO-konforme Speicherung der Daten
- Nachweis über Datensicherheit durch anerkannte Cloud-Computing-Zertifikate, z. B. „TrustedCloud“ des Bundesministeriums für Wirtschaft- und Klimaschutz
- Diverse Verschlüsselungs- und Anonymisierungsoptionen bei Azure oder AWS
Pre-trained Models für jede Datengröße
Auch wenn Big Data häufig in einem Atemzug mit <a href="https://www.taod.de/services/data-engineering-consulting“ data-webtrackingID="blog_content_link" > Cloud Services </a> genannt wird, sind Data-Science-Projekte in der Cloud insbesondere auch für Use Cases interessant, bei denen wenige bis gar keine Daten vorhanden sind. Für solche Fälle stellt beispielsweise Azure Cognitive Services bereits standardisierte, mit eigenen Daten vortrainierte Modelle zur Verfügung, die mit minimalem Aufwand in das eigene Projekt integriert werden können.
Sollen etwa Textdokumente zur Sentiment-Analyse mittels einer Spracherkennungs-KI eingelesen werden, müssen nicht extra aufwändige NLP-Modelle durch per Hand digitalisierte Texte trainiert werden. Stattdessen können frei verfügbare Module wie Azure Cognitive Services in den Workflow eingebaut werden, die bereits über ein eigenes Vokabular verfügen. Aber auch, wenn bereits größere Datenmengen vorliegen, sind pre-trained Models nützlich, da – statt bei Null zu beginnen – Zeit und Daten effizient für die Weiterentwicklung des spezifischen Use Cases eingesetzt werden können.
Vom MVP zur Produktion in wenigen Klicks
Steht am Ende der cloudbasierten Data Science MVP-Entwicklung ein vielversprechendes Produkt, ist die Integration in das operative Geschäft deutlich einfacher, als bei einer lokalen Entwicklung. Dank der Entwicklung in der Cloud steht kein komplexer Umzug von der Entwicklungs- auf die Produktivumgebung an und Data Pipelines müssen nicht angepasst werden. Services wie AzureML wurden so entwickelt, dass MLOps mit dem geringstmöglichen Aufwand erfolgen kann. Das bedeutet, dass die Schnittstelle zwischen Machine Learning (ML) auf der einen Seite und operativem Geschäft (Ops) auf der anderen durch Funktionen wie automatische Bereitstellungs-Pipelines, Versionskontrolle und erweitertes Monitoring optimal integriert ist.
Das erleichtert nicht nur die Arbeit der Data Scientists, sondern ermöglicht auch die belastbare Nutzung des Algorithmus/Modells. Entscheidungstragende im Unternehmen können sich also bereits zu einem frühen Zeitpunkt der Entwicklung auf die gewonnenen Erkenntnisse bei ihrer Entscheidungsfindung im Alltag verlassen – dank Machine Learning und Data Science in der Cloud.