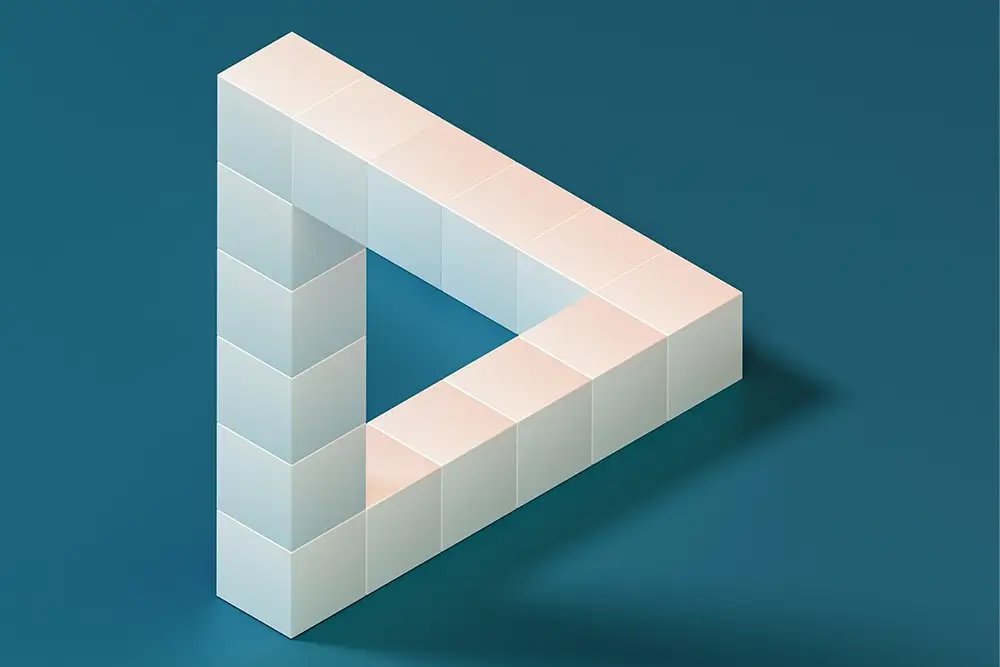
Reverse ETL: key component in the Modern Data Stack
A data warehouse enables the elimination of data silos, but can ironically become one itself. The collected data often lies around uselessly in the warehouse because its further use presents companies with a major challenge. The integration of reverse ETL pipelines is helpful.
Modern companies store huge amounts of transaction and analysis data in data warehouses such as Snowflake, Google BigQuery, Amazon Redshift or Azure Synapse. The data warehouse has become the central nervous system for a company and functions as a single source of truth in which all data is brought together in a secure and organized manner. By investing in a data warehouse, companies are forcing the elimination of data silos, which are known to be a blockade to efficient and powerful corporate alignment.
After all, if important data is not centralized in one place, but is incomplete and distributed in various tools, departments will end up working with different information and perspectives. Reverse ETL pipelines are used to integrate data from the data warehouse into the tools and processes used by employees on a daily basis in order to exploit its full potential.
Data integration approaches: The derivation of reverse ETL
To understand reverse ETL, it is helpful to take a look at the ETL and ELT integration approaches.
ETL refers to the sequence of classic data pipelines: Extraction - Transform - Load. Raw data is retrieved, transformed and fed into a database. The process of transformation refers to the cleansing, filtering, formatting, enriching and organizing of this data in order to simplify the creation of models in the database.
However, most cloud-based databases follow the modern ELT approach: Extract - Load - Transform. The data sources are transferred directly to the target system and only transformed there. This approach is particularly advantageous for large volumes of data, as it supports scalability and uses fewer resources.
Reverse ETL , on the other hand, refers to an integration approach in which current data is extracted from the data warehouse, transformed for further use and loaded into operational business applications or operational systems, unlike the approaches described above.
Why should data be moved from the warehouse if it is already stored there?
Reverse ETL enables companies to operationalize data in the systems and processes used and to take meaningful action with verified and trustworthy data. According to the "close the loop" principle, relevant and useful findings are obtained and then used and applied in a targeted manner. In addition, a company-wide standardized definition of core metrics can be ensured. The advantages of reverse ETL are
Data automation
Workflows can be automated and transferred directly within the operating system. Reverse ETL enables the efficient and optimized creation of workflows and the elimination of time-consuming manual requests for data.
Reduction of cross-team data silos
A verified database facilitates cross-departmental collaboration and avoids errors. Data required for decisions and strategies does not have to be searched for, but can be integrated directly into the appropriate tools and systems.
Increasing customer satisfaction
Reverse ETL can improve the customer experience and have a positive impact on sales. The customer experience is personalized using existing data from a customer data platform to find simple solutions to problems that customers may be facing. In addition, personalized marketing campaigns can be played out to potential customers and expand the customer base.
The role of reverse ETL in the modern data stack
Reverse ETL is an elementary component for the adequate preparation of data within the Modern Data Stack. The Modern Data Stack is a multi-layered system of modular tools that fully process a company's data, from the connection of data sources to data storage and data visualization. This system follows the principle of reliably filtering data along the digital value chain in order to be able to use it strategically, scalably and efficiently. Ideally, data management follows the following principle:
Step 1: Acquisition
As explained at the beginning, investing in a cloud data warehouse as a single source of truth is essential for modern and data-driven companies. This primarily serves as a central storage location for all data that originates from different sources.
Step 2: Preparation
Before the collected data can be used, it must be made usable for various systems. This is where reverse ETL pipelines come into play, closing gaps and integrating data from the data warehouse into target systems. Transformations and modeling prepare the data so that its format, values and properties are optimally prepared for analysis.
Step 3: Analysis
In order to fully exploit the value of the data, an analysis process including the creation of visualizations should be established. This makes it possible to derive insights, discover correlations and create forecasts.
Reverse ETL for agile data warehouse management
A bidirectional ETL approach makes it possible to integrate data and the insights gained from it more quickly within the company and in the tools used on a daily basis and to place them with decision-makers. Accordingly, reverse ETL tools are key components of the modern data stack, avoid the creation of data silos in the data warehouse, automate manual data queries and increase efficiency. In addition, updated data can ensure a more seamless and personalized customer experience and thus increase customer satisfaction.