
Ready for a new era
It has long been possible to use artificial intelligence (AI) within a company. Nowadays, this option is becoming more and more of a necessity. But what exactly is AI, how can companies use it and where does it make sense? Dario Luipers from the Mittelstand-Digital Zentrum Rheinland provides answers in this guest article.
Thanks to the latest achievements in AI research and development, a number of AI tools are available that can be integrated into everyday working life at any time. AI-based language applications, which are based on so-called Large Language Models (LLM), automatically generate texts based on questions or key points, shorten them or formulate the content in the desired language style. AI-generated texts can even be used for initial customer consultations. A current example of this is ChatGPT, which many companies are currently implementing as a professional text creation tool. AI-based image applications, such as Stable Difusion, are used to create new image material. Companies like to use such visual innovations for advertising purposes or to create presentations.
From a value creation perspective, data-driven analyses and process support are the far more sensible areas of application for AI, especially in the production sector. Of course, this requires data that is used to teach machine learning algorithms to recognize patterns in the data structures. What is generally referred to as AI are usually so-called deep learning models or deep neural networks, which are modeled on the neuronal connections in brains. In some cases, however, simpler algorithms, such as linear regression, can also be used. The goal of these algorithms determines the area of application.
Train machine learning models
A distinction can be made between regressions, classifications and clustering. Regressions, or predictions, can be applied to different scenarios. These include predictive maintenance, which can forecast machine downtimes, or, in the case of predictive quality, the product quality of the component to be produced. Classification is increasingly being used in the automatic inspection of components using machine vision algorithms. In the case of clustering, the algorithm attempts to form data categories. For example, combinations of machine parameters that lead to increased rejects can be identified. All of these applications require well-trained machine learning models. In this case, "good" means that the statements, or the conclusions drawn from the input data, are consistent. There are two criteria for achieving this: Data quantity and data quality
The quantity of data for teaching AI should be as high as possible. The more the better is the motto here. The greater the variation in the data basis, the more different examples are available for the algorithm to learn from. It is important that no outdated data is used that may no longer reflect the current status of the process. This aspect is directly linked to the topic of data quality. The data from which the algorithm learns should be as "clean" as possible. This means that there are no gaps in the data recording due to failed sensors, for example. The data must not be too "noisy". The standard deviation is a good indicator here; it should not be too high. Outliers from the training data are best removed. Non-scalar values should be defined uniformly. This is the case, for example, when evaluating product quality.
Standardized procedure
If all these parameters are correct, it should be possible to train a machine or deep learning algorithm on this data. It is worthwhile for the company to proceed methodically in order to use AI in a truly targeted manner. AI models can help here, as they provide concepts for the targeted implementation of AI in the company. The CRISP-DM(CRoss Industry Standard Processfor Data Mining), a standardized process model for data mining projects, is recommended. CRISP-DM allows the individual work steps to be precisely defined. The main phases are summarized below.

Business Understanding
AI is not an end in itself, but must be checked for the corresponding use case before it is used.
Data Preparation
This step ensures data quantity and quality. It requires the most work capacity and forms the basis for a functioning AI model.
Evaluation
After the AI model has been trained, the results of the model must be examined in detail. Does the model not produce the expected results? What could be the reason for this? By answering this question, important insights can be gained about the process that is to be supported by the AI.
Deployment
If the previous steps were successful, the next step is to put the AI model into use. It is important to record this process in easily understandable and concise documentation. This also includes the new AI-supported process that is now in place. This must be maintained and lived.

People and processes
From the time of deployment, the process must be maintained and serviced. The algorithm must now be continuously supplied with data. A corresponding data pipeline must be implemented for this purpose. This ensures that the algorithm receives data in real time, for example from a machine, in order to be able to make predictions about product quality or the time of maintenance. Just as with AI training, the data must be of high quality. Incorrect values or faults in the data acquisition process render the expensively implemented algorithm useless. The AI system must be regularly retrained with new data. Otherwise, fluctuations in the process will no longer be interpreted correctly by the algorithm at some point. The results of the AI should be evaluated regularly in order to identify problems in the process at an early stage.
The human factor should also not be forgotten. Ultimately, processes are still implemented and lived by people. It is therefore important to bring those responsible for AI projects and the employees on site together at an early stage and to consider all interests and aspects that affect the process to be optimized by AI.
One of the projects implemented, in which the author of this article was involved, involved monitoring the painting of a component using AI-supported image recognition. Defective painted areas were to be identified and thus rework or rejects automatically detected. The steps outlined were all carefully implemented. Shortly after implementation, each component was marked as a reject. The reason for this was the change in position of the installed camera for data recording. Employees felt that their work was being observed, even if the task area was not directly related to quality control in the painting area and the camera system was not filming a human work area.
AI and communication
This experience shows how important early communication is in AI projects. The goal, as well as the affected work areas and processes, must ideally be communicated with the entire workforce. Fears, such as the loss of a job, must be taken seriously and discussed. In most cases, these are unfounded or the company is generally at risk because it does not prioritize the topic of AI and data generation and utilization (AI readiness) sufficiently.
AI will have to become an integral part of every company. In order to be able to use AI effectively, data of a high quantity and quality is the basic building block. In addition, an infrastructure must be in place to record and store data in real time during ongoing operations. From this point onwards, enormous added value can usually be created for companies even without AI, purely through analysis. All of this is often not possible without external help. In addition to many service providers on the market, there is also provider-neutral and free initial support through funded projects. One of these is the Mittelstand-Digital Zentrum, which provides initial assistance in the field of AI thanks to funding from the Federal Ministry for Economic Affairs and Climate Protection.

This article first appeared in a modified form in issue 02/23 of our magazine data! You can find all issues and articles here:
data! Magazine: Cloud Services, Data Analytics & AI | taod
About Dario Luipers
Dario Luipers heads the Mittelstand-Digital Zentrum Rheinland and has been working on artificial intelligence and big data topics since his studies.
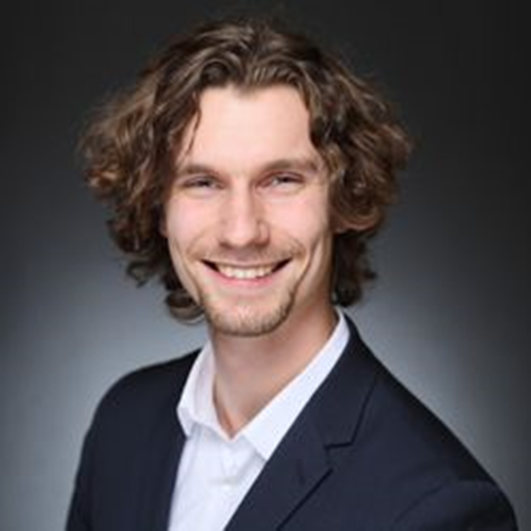