
Data analysis with special challenges
Project management and project implementation play a special role in exploiting the full potential of data science in the company. When answering questions using methods from the field of data science or machine learning, it is important to be aware of their specific requirements. This is the only way to ensure a successful data science project.
The challenges of data science projects lie on the one hand in their complexity, and on the other in the precarious predictability of time and resources to achieve a specific goal.
Special features of data science projects
In addition to the research question, the existing data basis is essential for implementation. It is not only data preparation that plays a role here, as new features usually have to be modeled before the algorithms can be applied. It is also quite possible that a data set does not yet contain sufficient information for some aspects of the research question. In this case, the starting points for the project are recalibrated. This uncertainty should always be taken into account in a data science project.
In order to be able to provide the necessary specialist knowledge of the issue to be investigated, a constant exchange with customers is a basic prerequisite and recommended for the creation of a precise model. This is because data often depicts processes that require knowledge of their interrelationships in order to create meaningful derivations.
For these reasons, a special approach is required for a data science project in order to meet the emerging challenges flexibly.
As everywhere else, the same applies to data science: ask questions!
Everything always starts with asking questions. These can be as follows:
- How will my business develop in the future?
- What causes customers to cancel my service or stop ordering my product?
- Are there customer groups that exhibit similar behavior so that I could target them more effectively with personalized advertising?
- How many products should I keep in stock next month to cover my requirements?
- How can I optimize my processes?
Depending on the company, a wide variety of questions arise and this list of questions could go on forever. However, very few companies have sufficient resources in the area of data science. In order to guarantee a successful project approach, it is therefore advisable to work with external data analysts.
Identify use cases
Evaluating whether the questions that have arisen in the company can generally be answered using suitable machine learning algorithms is the first step in the joint collaboration. It may even be possible to read answers to questions from the data with very little effort. Complex analyses may then not even be necessary. A personal meeting or workshop with a data scientist is suitable for this.
The data scientist looks at the issues here from a method-oriented perspective. This means that his or her knowledge of the available algorithms or processes and his or her statistical knowledge come into play. On the other hand, the data scientist, together with the customer, must also determine the relevance of the questions and the effort required to answer them from a business perspective for future business success.
Cost-benefit calculation of data science projects
Of course, project costing is an important point: How complex will the project be? And is my data even suitable for creating precise models?
The expected scope of a data science project depends heavily on which data is to be used. And how precise the model should be as a result, as well as many other factors that only allow an estimate of the scope if the project parameters are known.
Once one or more relevant questions have been identified, it is necessary to assess their feasibility using the data collected by the company. This is because not every data set is suitable for the application of the methods and not every data basis is sufficient for every question. Perhaps the data is suitable in principle, but relevant steps still need to be taken for data processing. Ideally, the entire data should be evaluated with regard to the research question.
However, this approach often proves difficult, as not every company is prepared to make its data available to an external service provider. The good news here is that representative sample data is often sufficient to assess feasibility. This means that such sample data can often be used to create an initial model and estimate the cost of project implementation. This approach enables a realistic estimate of the effort, time and resources required and is a good basis for a successful project.
Procedure for data science projects
The iterative approach to data science projects has established itself as the standard. If a method used does not yet deliver the desired accuracy, this can have many different causes. It is also quite normal at the beginning of such a project. The algorithm used may not be suitable for the specifics of the data at hand. For this reason, different methods are often used to create models and their accuracy is compared. In addition, the parameters used for the methods can be optimized.
Decisive improvements can often be achieved simply by working closely with the customer, who can pass on their specialist knowledge of the problem to the data scientist. The data scientist can then use the newly acquired information when creating the model in order to use certain features or generate new ones and then feed them into the algorithm. Here, the data scientist must demonstrate creativity, domain knowledge, a feel for data and knowledge of the algorithms.
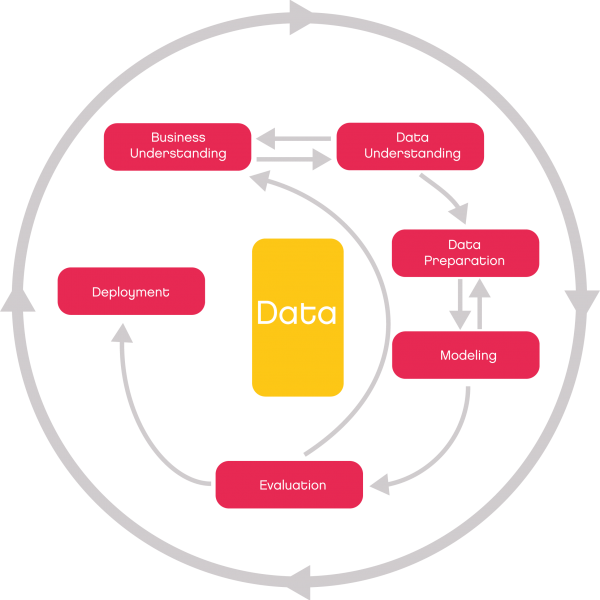
Advantages of the agile approach to data science
Due to the special requirements, an agile approach is particularly suitable for data science projects. In an agile approach, interim goals are defined at the beginning of an iterative cycle. The results from these interim goals serve as the basis for the next objective in the project. This allows cost and resource budgets to be flexibly adapted to the course of the project.
This makes sense as the project develops gradually. Requirements change, new data sources may become available or new questions may arise from the knowledge already gained. If the project team identifies significant additional work at an early stage of the project, it can react flexibly and change the course of the project without incurring unnecessary costs or resource bottlenecks. An agile data science project of this kind therefore begins with an initial basic model, which is then gradually optimized as the project progresses and moves on to the main phase of the project, which is characterized by the achievement of milestones.
Our experience shows that the agile approach contributes to an increase in efficiency, better cooperation with the customer and higher customer satisfaction. In many waterfall-style projects, the lack of communication between those involved leads to a divergence between expectations and results over the course of the project. The agile approach allows companies to respond to both foreseeable and unforeseeable requirements in an appropriate time and quality and to achieve convincing results.
The PPP rule: plan, test, perfect
Due to the initial planning uncertainty, every data science project requires a special feel for the project parameters at the beginning. It depends on a dedicated question and an initial assessment of whether the available data basis allows such an answer.
The data or a representative part of the data must definitely be checked at the start of the project. As it forms the basis for the algorithms applied to it, data quality must be a basic requirement in order to be able to make valid cost estimates.
At the same time, during the course of the project it is essential to drive forward the specification of the parameters through constant communication with the customer. This is the only way to ultimately create a precise model. This is only possible with an iterative and agile approach. After all, findings in data analysis are not just the end product, but are generated continuously throughout the process.